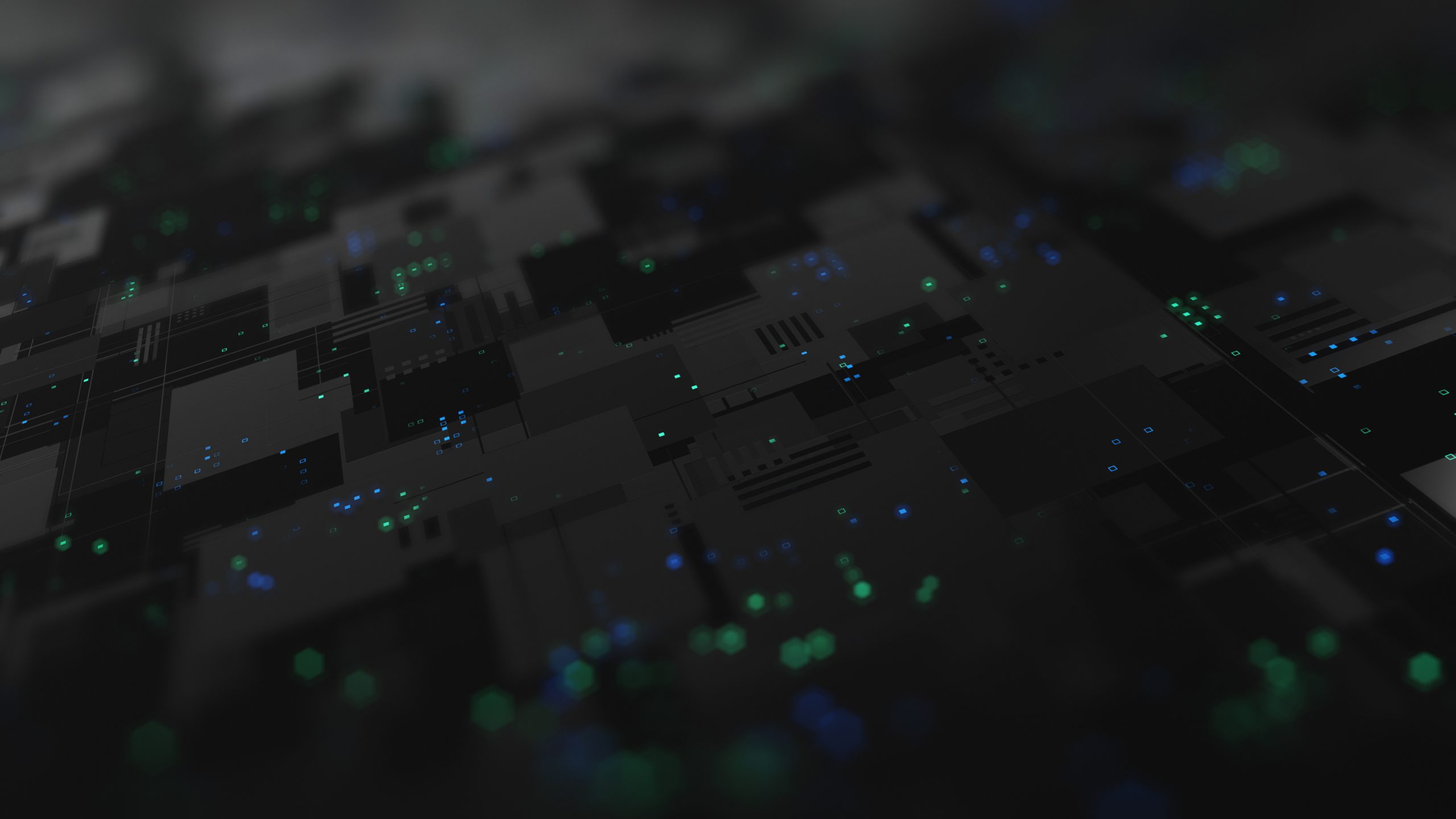
How Evolution Generates Complexity, Diversity and Intelligence?
The Power of Evolving Machine Intelligence
About EMI Group
We are actively recruiting Postdocs and RAs. If you are interested in joining us, please drop an email to: ranchengcn@gmail.com (Dr. Ran Cheng).
The Evolving Machine Intelligence (EMI) group was founded in September 2018, affiliated with Southern University of Science and Technology (SUSTech), China. Generally, our research motivations can be interpreted at three levels:
- Philosophically, we are motivated to study the evolvability of intelligence;
- Scientifically, we are motivated to understand how evolution generates complexity, diversity and intelligence;
- Technically, we are motivated to design learning/optimization computing paradigm across areas between evolutionary computation and deep learning, reinforcement learning, operation research, etc.
Our cutting-edge research outcomes provide full-stack solutions across hardware devices, algorithms, systems and applications.
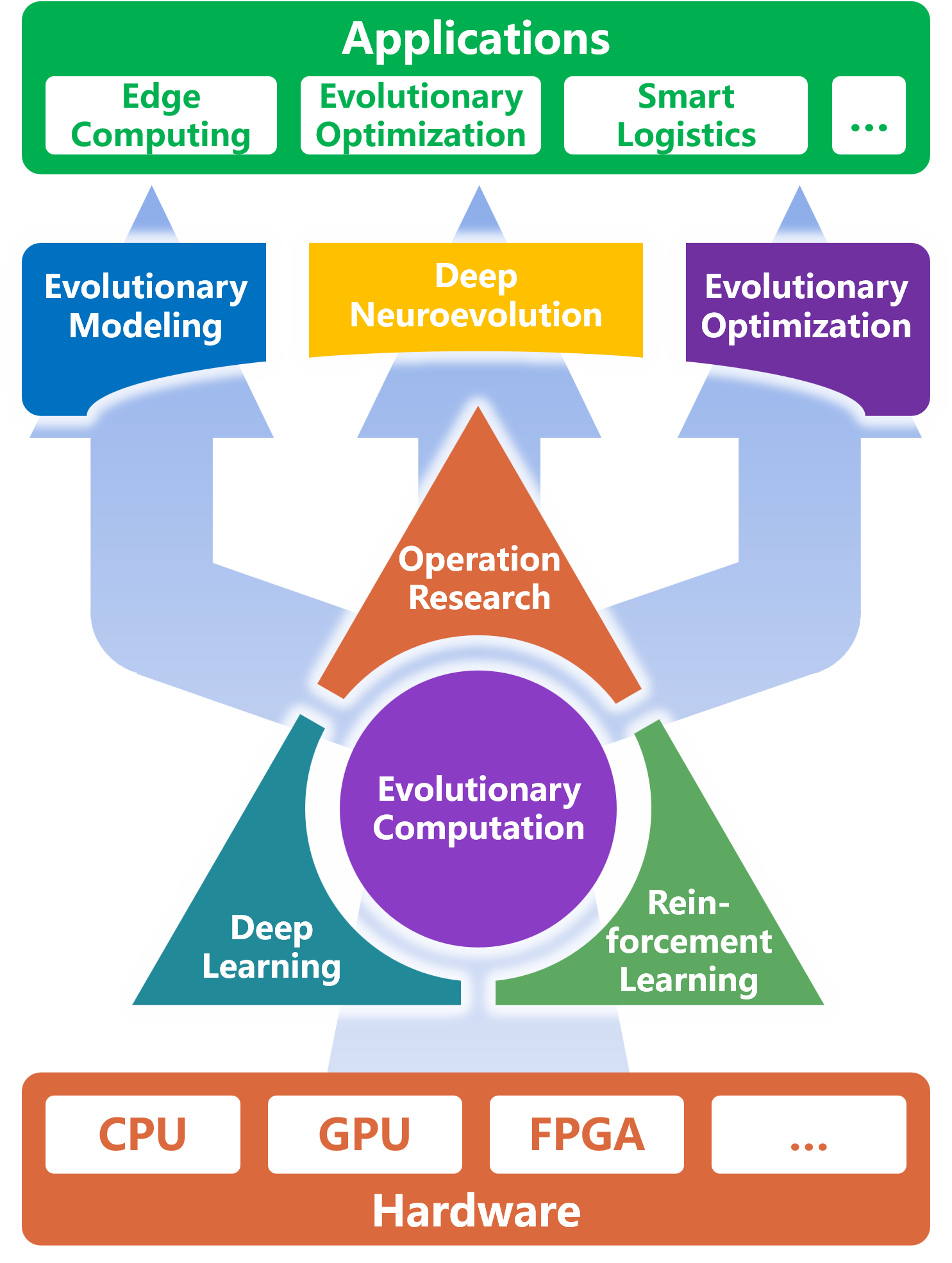
Deep Neuroevolution

Evolutionary Optimization

Evolutionary Modeling
