Yiming Chen, et al.
Abstract:
As unlabeled data carry rich task-relevant information, they are proven useful for few-shot learning of language model. The question is how to effectively make use of such data. In this work, we revisit the self-training technique for language model fine-tuning and present a state-of-the-art prompt-based few-shot learner, SFLM. Given two views of a text sample via weak and strong augmentation techniques, SFLM generates a pseudo label on the weakly augmented version. Then, the model predicts the same pseudo label when fine-tuned with the strongly augmented version. This simple approach is shown to outperform other state-of-the-art supervised and semi-supervised counterparts on six sentence classification and six sentence-pair classification benchmarking tasks. In addition, SFLM only relies on a few in-domain unlabeled data. We conduct a comprehensive analysis to demonstrate the robustness of our proposed approach under various settings, including augmentation techniques, model scale, and few-shot knowledge transfer across tasks.
Results:
Main Results
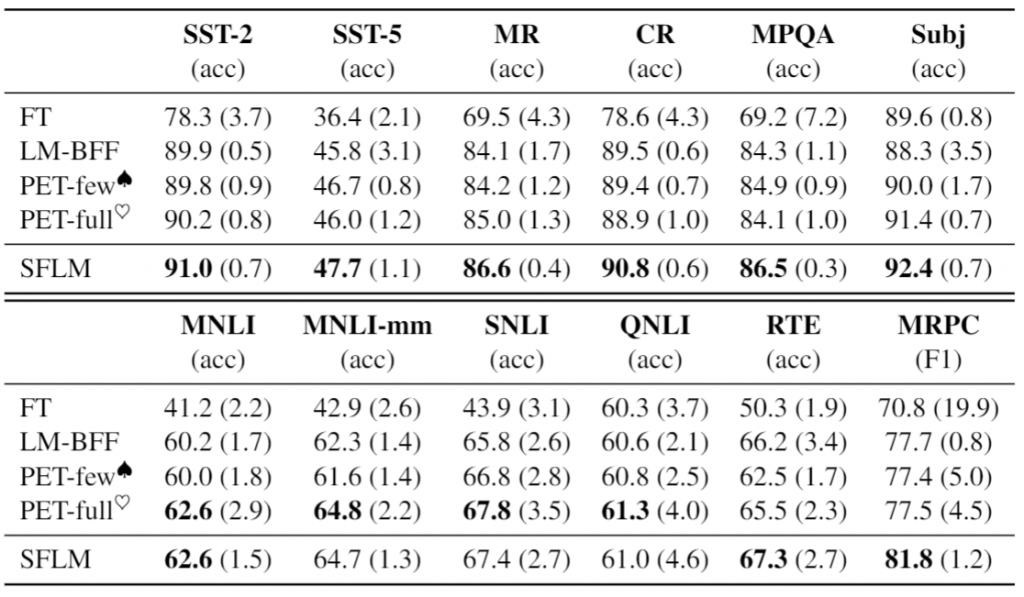
Data Efficiency Analysis
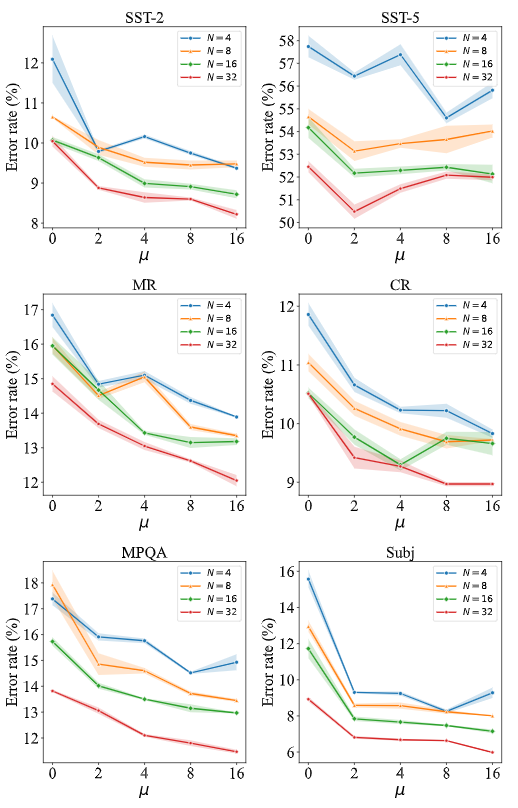
Augmentation Analysis
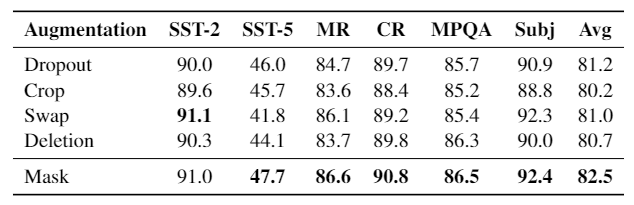
Model Scale Analysis
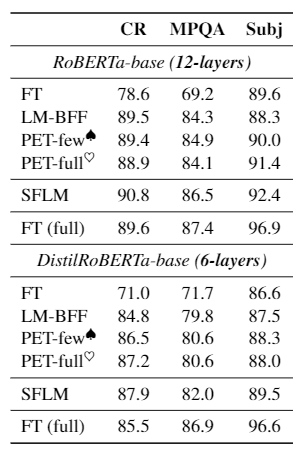
Citation:
@article{chen2021sflm,
title={Revisiting Self-Training for Few-Shot Learning of Language Model},
author={Chen, Yiming and Zhang, Yan and Zhang, Chen and Lee, Grandee and Cheng, Ran and Li, Haizhou},
conference={EMNLP},
year={2021}
}
Acknowledgments:
This work is partly supported by Human-Robot Interaction Phase 1 (Grant No. 19225 00054), National Re-search Foundation (NRF) Singapore under the National Robotics Programme; Human Robot Collaborative AI for AME (Grant No. A18A2b0046), NRF Singapore; National Natural Science Foundation of China (Grant NO. 61903178, 61906081, and U20A20306); Program for Guangdong Introducing Innovative and Entrepreneurial Teams (Grant No. 2017ZT03X386); Program for University Key Laboratory of Guangdong Province (Grant No. 2017KSYS008)