Recently, the EMI research group has made progress in the research field of large-scale multi-objective optimization. The result is entitled “Paired Offspring Generation for Constrained Large-scale Multiobjective Optimization” and published in the famous academic journal “IEEE Transactions on Evolutionary Computation” (IEEE TEVC, impact factor: 11.169).
In recent years, with the in-depth development of intelligence in the industrial field, the current situation that can be solved such as the optimization of intelligent model parameters and high-precision industrial design has become increasingly prominent. The difficulty of this type of problem is reflected in the huge scale of variables involved, complex constraint conditions, and a large number of black box targets, which are difficult to solve by traditional optimization methods. This work reports an evolutionary multi-objective optimization method based on the generation of paired offspring, which achieves an effective solution to optimization problems with more than 100-dimensional decision variables, 10 goals, and complex constraint conditions. It has achieved a series of test problems. A robust result.
Researchers analyzed the pros and cons of existing large-scale evolutionary multi-objective optimization algorithms in solving unconstrained problems, and combined with traditional constraint processing methods, they proposed a new strategy for generation from offspring instead of environmental selection and improvement. This strategy makes full use of the advantages of infeasible solutions in promoting population convergence and pairing reproduction mechanism in maintaining diversity, analyzes the impact of different random pairing methods on the quality of progeny populations, and then balances constraint processing and large-scale decision variables/objectives The function processing provides technical guarantee for the research team to further solve the measurement error state evaluation of the voltage transformer of the power grid group.
The first author of this article is He Cheng, the research assistant professor of the EMI research group, and Cheng Ran, the associate professor of the EMI research group, is the corresponding author of the paper. The collaborators also include Kay Chen Tan (IEEE Fellow), Professor of Hong Kong Polytechnic University, and Yaochu Jin (IEEE Fellow), Professor of the University of Surrey, UK.
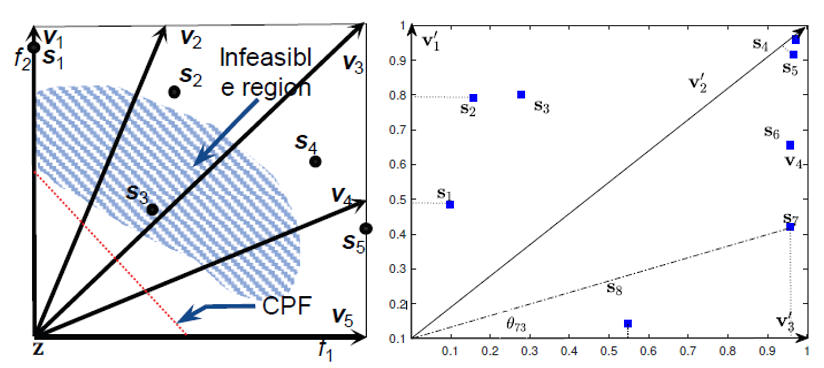
Fig.1 The target space subpopulation division method based on dual reference vectors, through the use of infeasible solutions and neighborhood relations, constructs generation methods for different constraint scenarios, and realizes algorithm constraint processing, convergence enhancement and diversity Keep the balance.
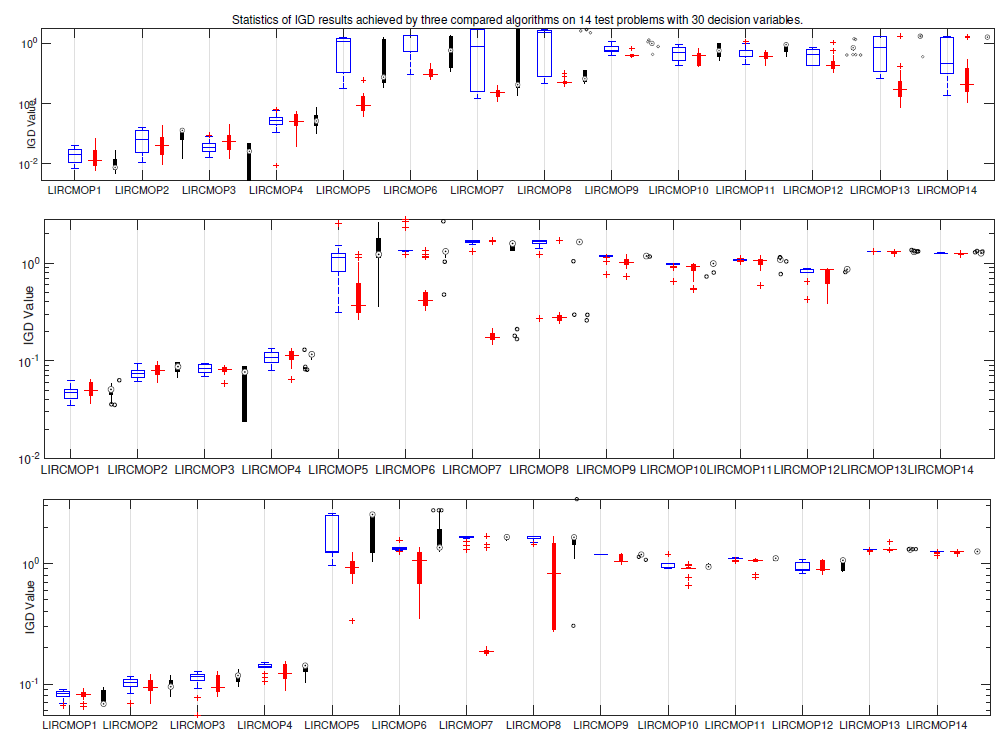
Fig. 2 The performance difference of different pairing methods of individuals under the same subpopulation in constrained large-scale multi-objective optimization problems. Deeply explored the influence of pure random pairing, repeatable random pairing and non-repeatable random combination on the performance of the algorithm.
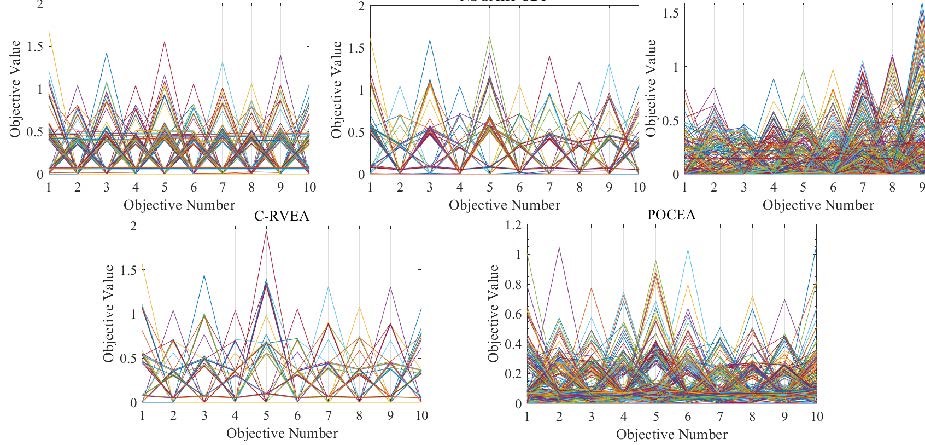
Fig.3 The results of different multi-objective constraint processing algorithms on the 200-dimensional 10-objective constraint optimization problem. The algorithm POCEA proposed in this work has achieved a final solution set with good convergence and uniform distribution on this problem.