Recently, the EMI research group has made progress in the research field of evolutionary reinforcement learning. The work is entitled “Lamarckian Platform: Pushing the Boundaries of Evolutionary Reinforcement Learning towards Asynchronous Commercial Games” and published in the famous academic journal “IEEE Transactions on Games” (IEEE ToG, impact factor: 1.237).
Reinforcement learning (RL), as a powerful tool for sequential decision-making, has achieved remarkable successes in a number of challenging tasks varying from board games, arcade games, robot control, scheduling problems to autonomous driving. However, when applying RL to real-world scenarios, there are also a number of technical challenges such as brittle convergence properties caused by sensitive hyperparameters, temporal credit assignment with long time horizons and sparse rewards, difficult credit assignments in multi-agent reinforcement learning, lack of diverse exploration, a set of conflicting objectives for rewards, etc. To meet the above challenges, recently, there has been an emerging progress in integrating RL with evolutionary computation (EC) to address the above challenges. While reinforcement learning enjoys the advances of several state-of-the-art platforms or frameworks, the literature is still in the absence of a platform or framework specifically tailored to evolutionary reinforcement learning (EvoRL). Moreover, current distributed platforms do not use computational resources efficiently, and thus the training of RL is extremely time-consuming for complex commercial games. Therefore, we introduce Lamarckian – an open-source platform featuring support for evolutionary reinforcement learning scalable to distributed computing resources, which shows unique advantages over the state-of-the-art RLlib with up to 6000 CPU cores.
Researchers study the status of existing frameworks or platforms, and especially investigate the pros and cons of the state-of-the-art RLlib and point out that RLlib is low-efficient in large-scale computational instances due to its computational mechanism. Besides, all the existing frameworks or platforms cannot support the asynchronous game environmental interfaces. Hence, to improve the training speed and data efficiency, Lamarckian adopts optimized communication methods and an asynchronous evolutionary reinforcement learning workflow. To meet the demand for an asynchronous interface by commercial games and various methods, Lamarckian tailors an asynchronous Markov Decision Process interface and designs an object-oriented software architecture with decoupled modules.
The first author of this article is Hui Bai, a Ph.D. student of the EMI research group, and Ran Cheng, the Associate Professor of the EMI research group, is the corresponding author of the paper. The collaborators also include Ruimin Shen, the researcher of the Game AI research team of NetEase Games AI Lab, Yue Lin, the director of NetEase Games AI Lab, and Botian Xu, the researcher at Institution of Interdisciplinary Information Science, Tsinghua University.
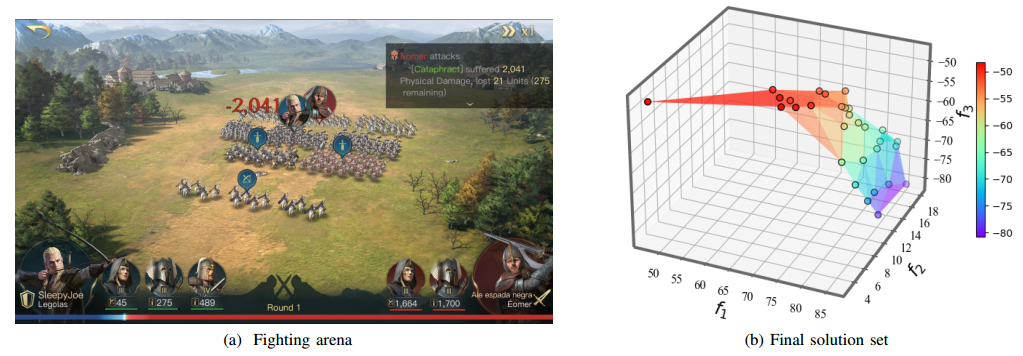
Fig.1. (a) is a typical hero-led fighting arena of The Lord of the Rings: Rise to War. (b) is the final solution set obtained by Lamarckian to a three-objective optimization problem in the game balancing test. f1 is the battle damage difference, f2 is the remaining economic resources multiples, and f3 is the strength of the weakest team.